Multimodal and Inclusive Language Models for General and Clinical French
The Pantagruel project (ANR 23-IAS1-0001) aims to develop and evaluate inclusive multimodal linguistic models (written, oral, pictograms) for French. It brings together researchers from various disciplines such as computer science, signal processing, sociology, and linguistics to ensure reliable and diverse results.
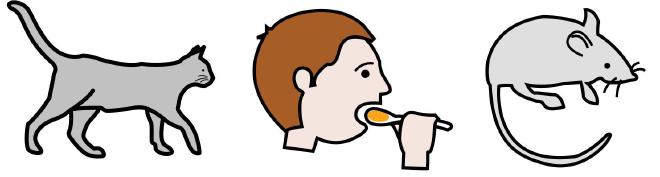
The main contributions aim to create self-supervised models accessible for French, adapted to different application domains. Additionally, the project plans to establish test benches to evaluate these models, leveraging previous experiences.
Particular attention is given to reducing biases and stereotypes in the data and models. Measures will be taken to mitigate these biases, considering the demographic characteristics of speakers and authors, with the support of an ethics committee.
The project also aims to develop software tools that facilitate the integration of these models into various applications, emphasizing accessibility for non-technical users.
Overall, Pantagruel seeks to improve multimodal linguistic models for French, with potential implications in various fields such as health and the arts.